INFO College Research Groups
At the University of Maryland College of Information Studies (INFO College), faculty, staff, and students are exploring how people access and use information. From developing smart city technology to creating new archival methods, we seek to improve the individual experience as well as to foster connected communities. Through our research centers, labs, and interest groups, we enable discovery, creativity, problem-solving, and fun while tackling real-world challenges and developing impactful solutions. All students are invited to participate in hands-on research and learning with our centers, labs and interest groups. Most groups also host public events bringing together thought leaders of the discipline.
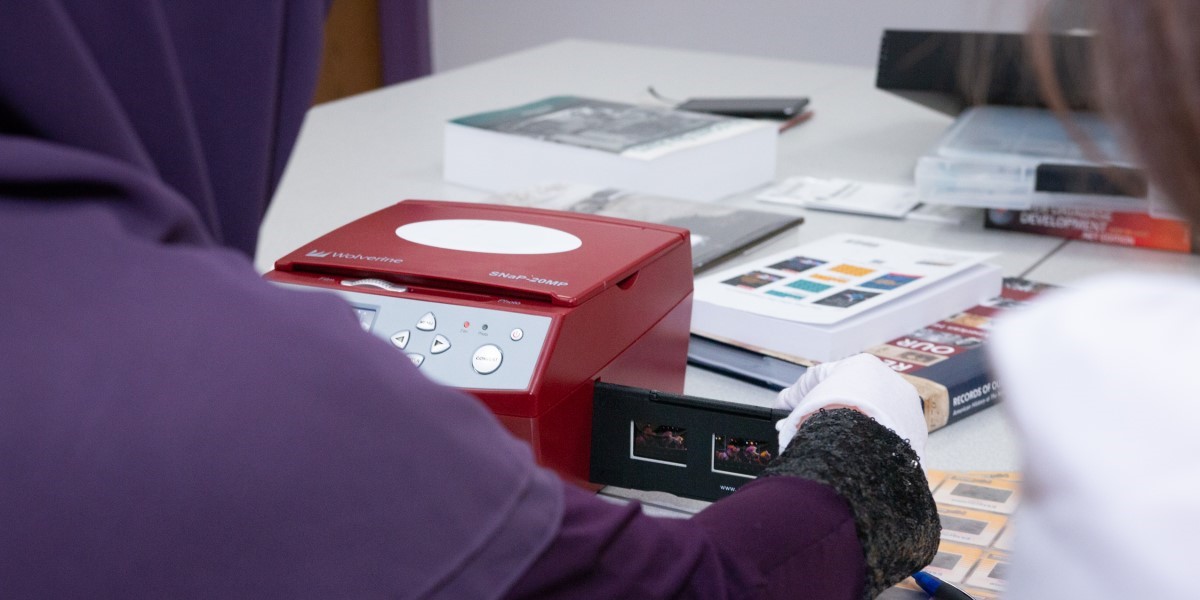
Center for Archival Futures (CAFe)
Creating systems to enable the use and care of digital objects and data over time.
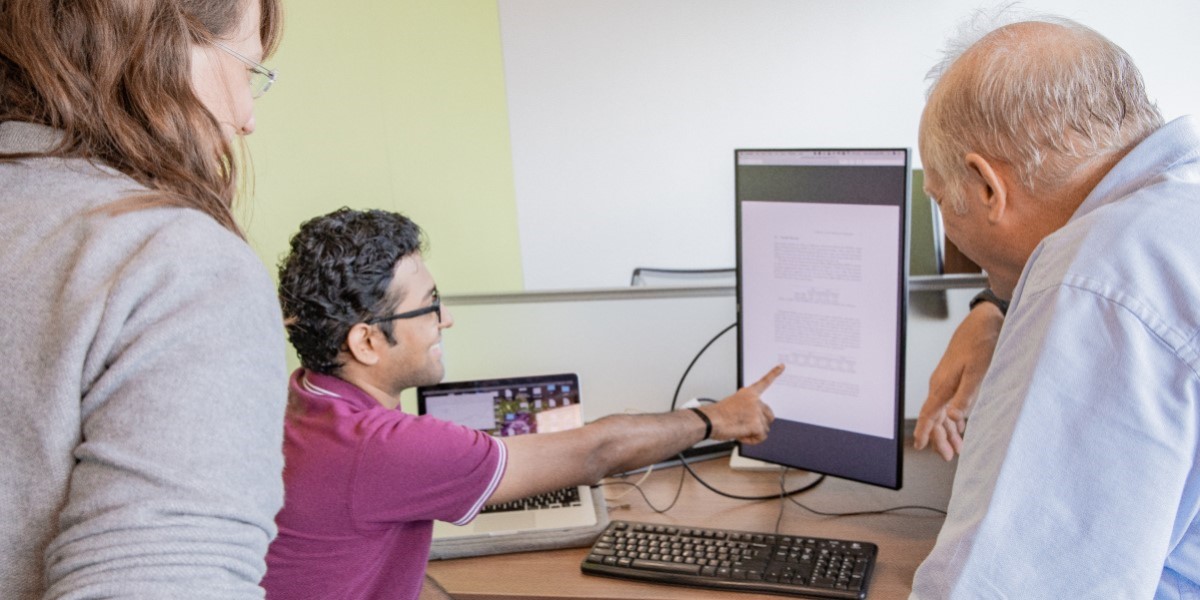
Computational Linguistics and Information Processing (CLIP) Lab
Designing algorithms and methods for computers to perform human language-related tasks.
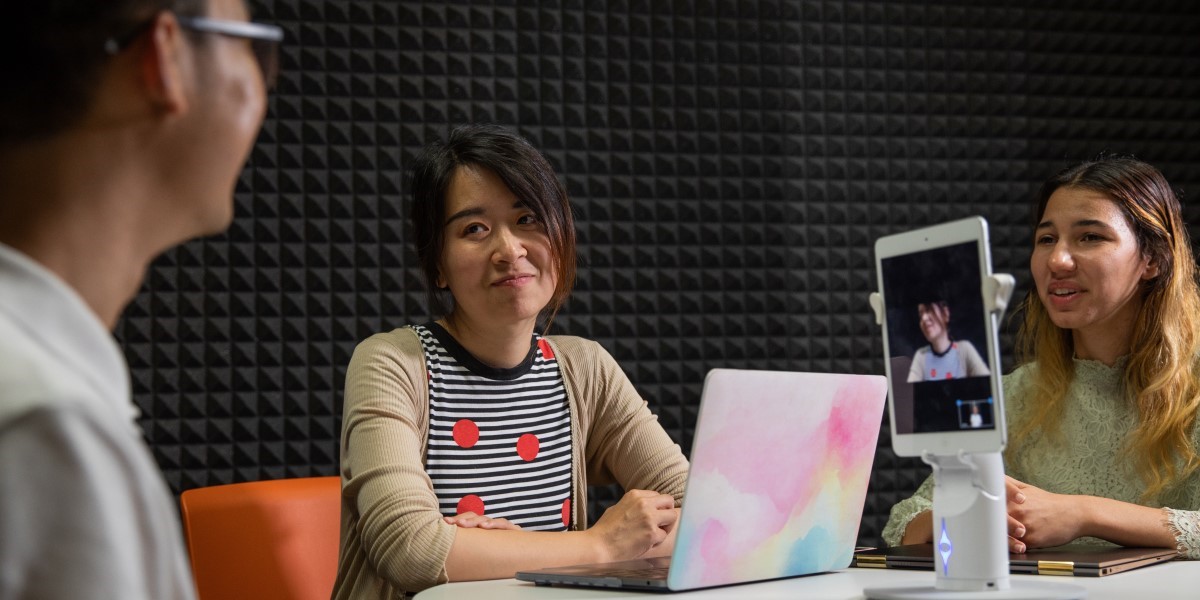
Human-Computer Interaction Lab (HCIL)
Transforming the experience people have interacting with technologies.
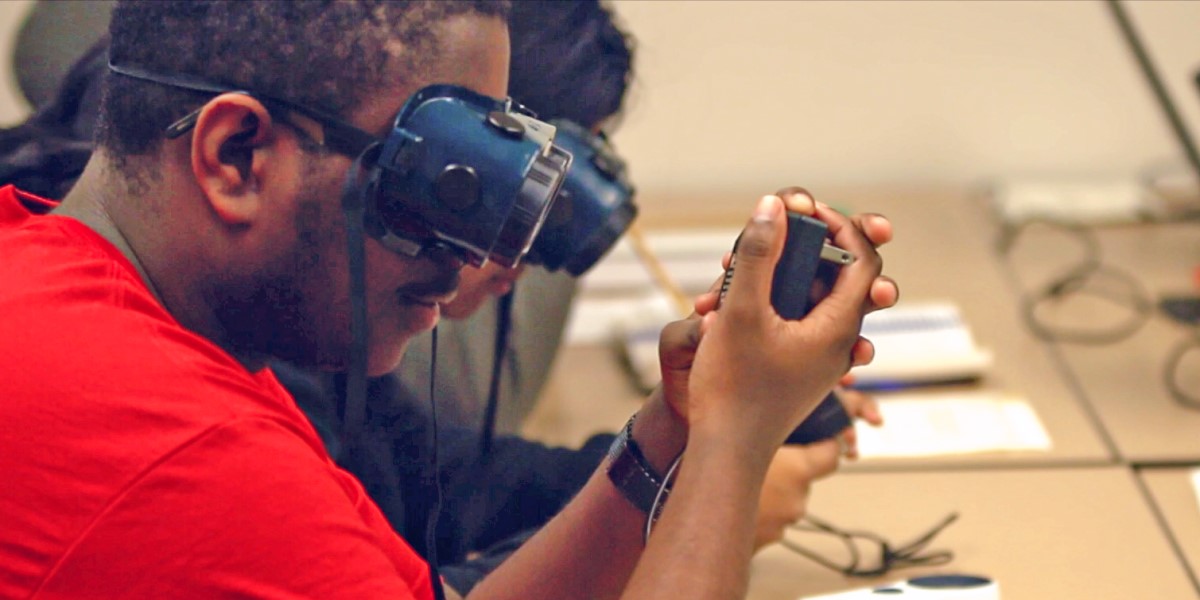
Maryland Initiative for Digital Accessibility (MIDA)
Collaborating with disability communities, tech companies, and policymakers to make digital technologies accessible for people with disabilities.
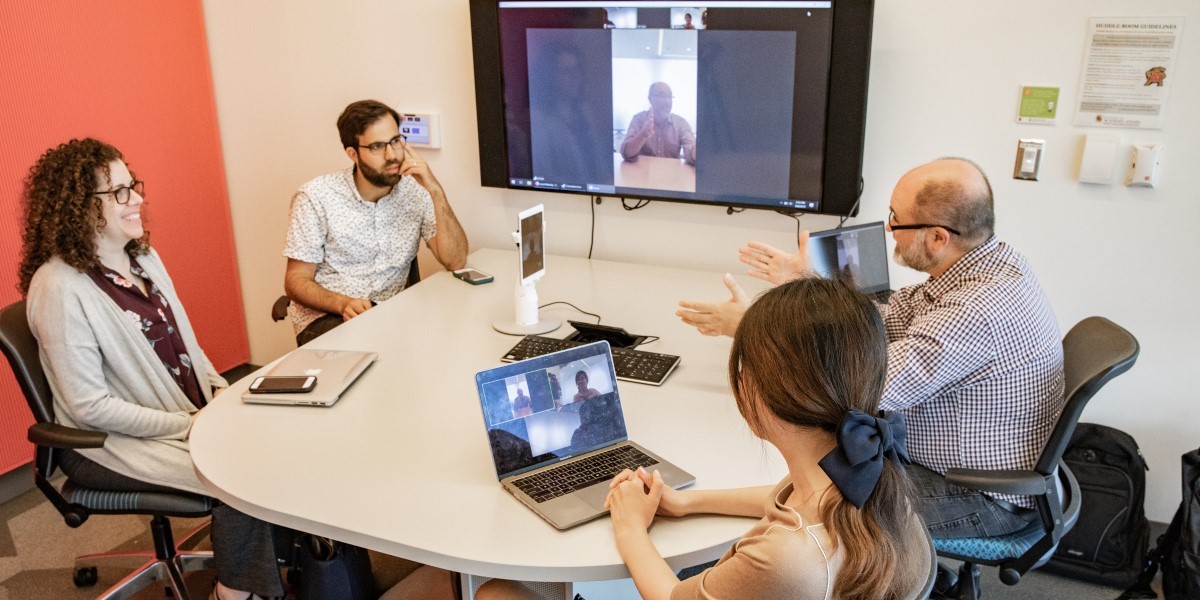
Organization Teams & Technology Society (OTTRS) Interest Group
Exploring the intersection between teams and technology with a focus on potential advances.
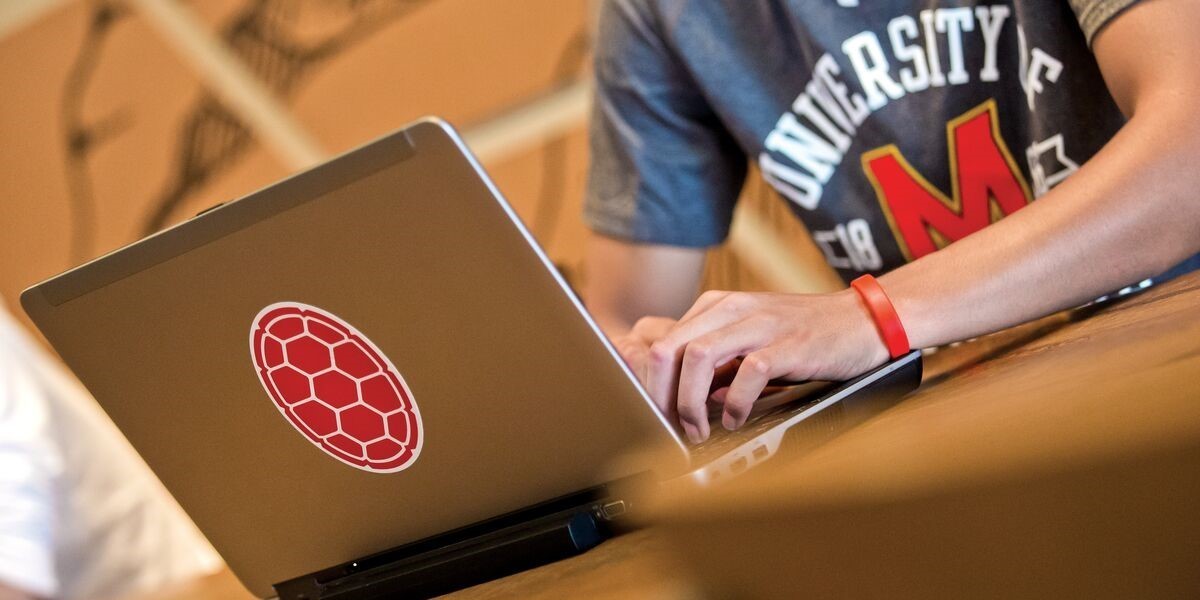
Search Mastery Interest Group
Advancing search literacy as a high-priority component of information literacy education.
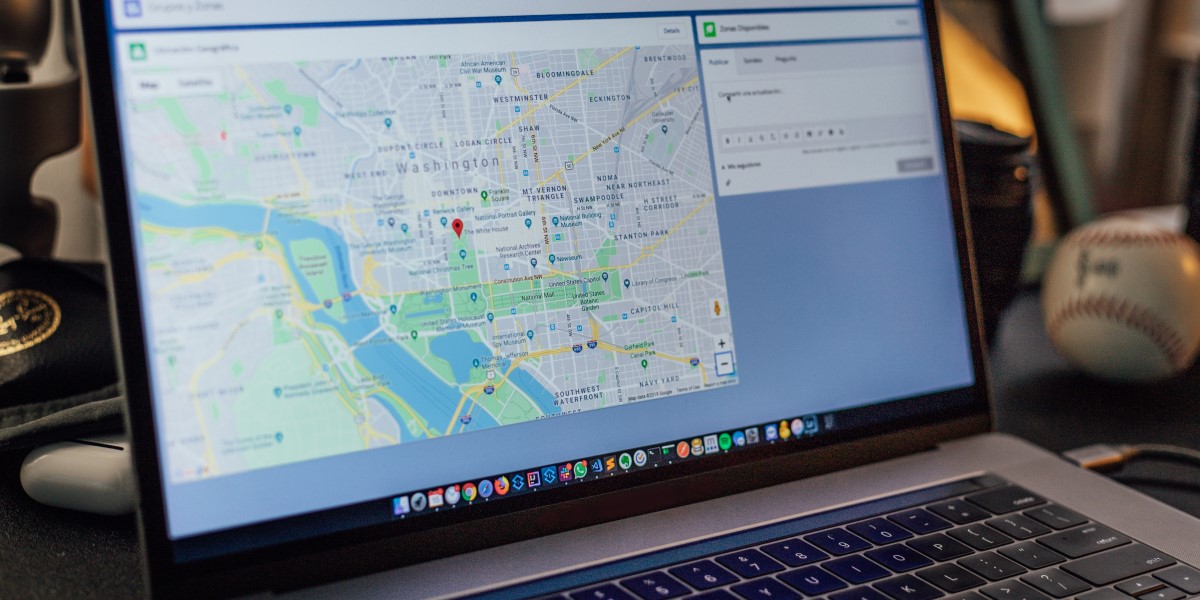
Social Data Science Center (SoDa)
Working with social and behavioral data at ever increasing scales for novel purposes.
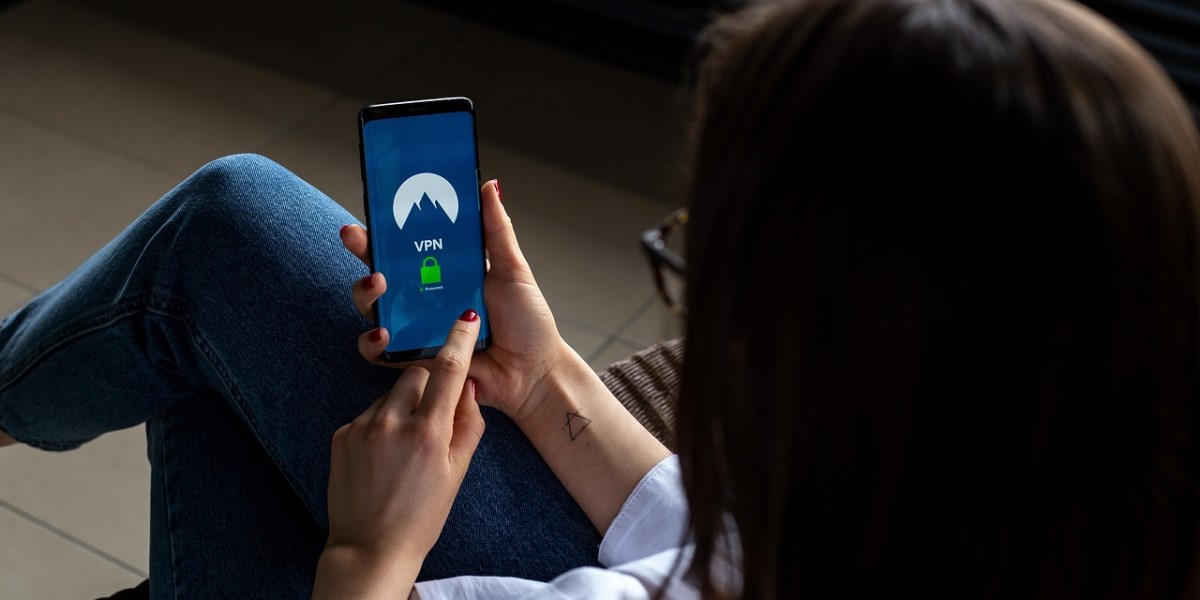
Sociotechnical Cybersecurity (STC) Interest Group
Examining and evolving current ideas around human aspects of cybersecurity.
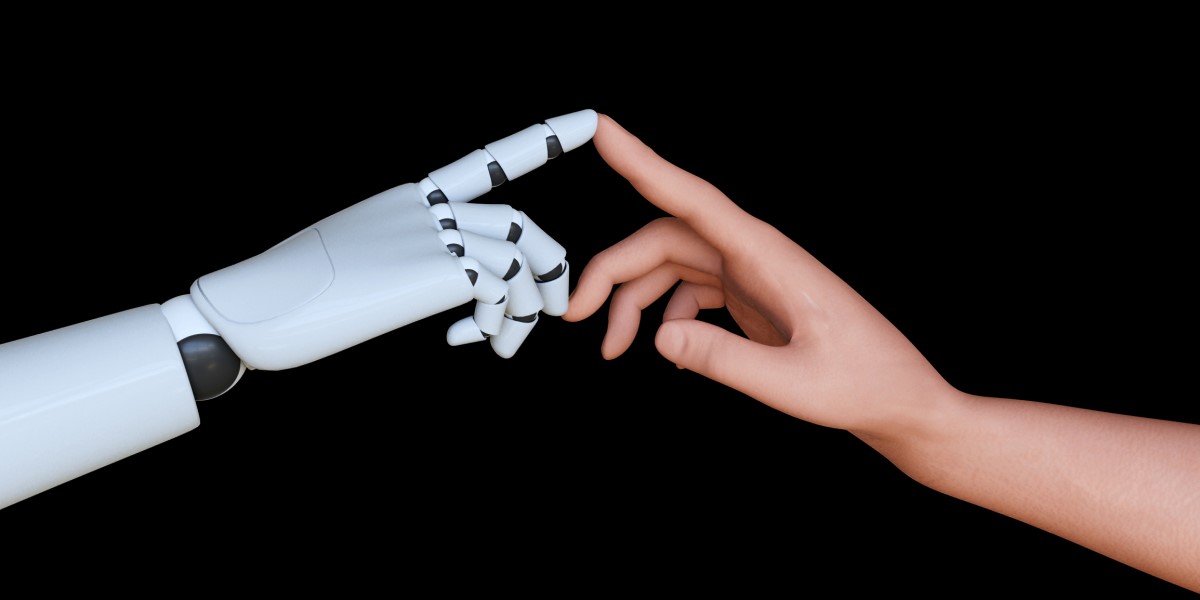
Values-Centered Artificial Intelligence (VCAI) Initiative
Integrating research and education across the UMD campus + connecting with high-impact stakeholders to transform how AI is practiced globally.